The use of drones, or unmanned aerial vehicles (UAVs), for agricultural applications is rapidly increasing. Unfortunately, the popularity of drones has taken some attention away from the enormous advances made in satellite-based systems in the last few years. I am continuously discovering new ways in which freely-available Sentinel-2 imagery can be used for agricultural applications. The main advantage of such imagery is that a new image of a particular production unit (e.g. camp, field, orchard, vineyard) is acquired every five days. Also, the images are acquired at exactly the same time of day, which means comparisons among image acquisitions are possible.
Apart from the obvious advantage of having five-day interval images of crops, one of the biggest advantages of satellite imagery is that one can generate a temporal profile for each production unit. For instance, Figure 1 shows the normalized difference vegetation index (NDVI) profiles extracted from Sentinel-2 images of three fields in Douglas, South Africa, which is a semi-arid region with a summer rainfall.
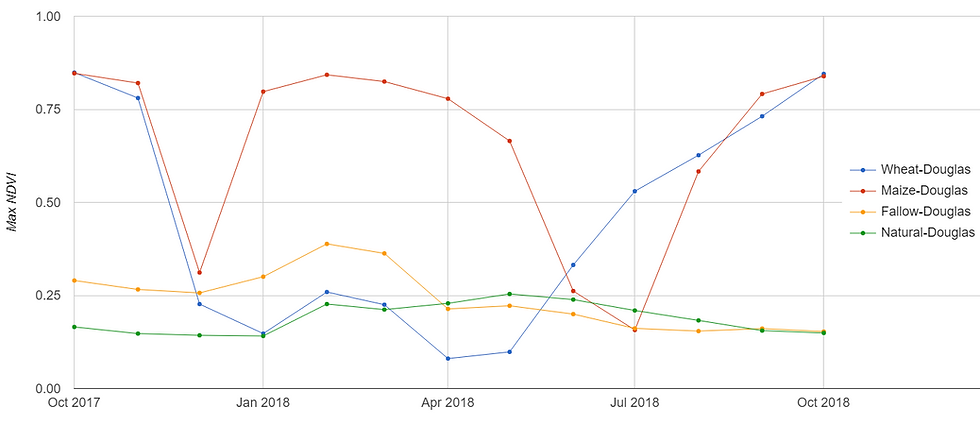
The Maize field was planted with maize (corn) in the December 2017 and with wheat in July 2018 (a practice known as double cropping). The Wheat field was left fallow during the summer 2017/18 season and planted with wheat in May 2018. The Fallow field was not planted at all and has a comparatively uniform NDVI profile for the period. Similarly, the profile of natural vegetation (Natural) (also sampled in Douglas) is relatively uniform compared to the Wheat and Maize fields. Both the Wheat and Maize fields were consequently irrigated.
Figure 2 shows the NDVI profiles for a rainfed Wheat field and an irrigated vineyard (Grapes) in Saron, South Africa. This area has a Mediterranean climate (winter rainfall and dry, warm summers). As in Figure 1, the profiles are compared to a fallow field and natural vegetation. Notice that the Wheat field in Saron is very different from the Wheat field in Douglas.

Satellite imagery allows for temporal profiles to be extracted over many seasons. Once they are extracted, they can be used in many ways. For instance, they can be used for benchmarking, i.e. if a production unit’s NDVI is higher than those of previous season’s, it is likely that the yield of that unit will be relatively higher. Coupled with yield data, the shape of the curves can also be used to make predictions about yield (e.g. in the Douglas region, a high NDVI at the end of the season has a bigger influence on yield than at the start of the season).
Another major application of these temporal profiles is crop type differentiation. By using machine learning, the temporal (phenology) variations among crops can be used to produce crop type maps over extensive areas. Such maps are critical for yield estimations at regional scales, which are used for commodity and future trading (because yields influence commodity prices).
Clearly there are enormous value in freely available satellite imagery, not only for identifying zones within production units that require closer inspection, but also for benchmarking production units against others and for estimating yields over large areas.
This article was originally published on www.remotesensing.blog, which is devoted to articles about the use of remote sensing and other geospatial technologies for agricultural and related applications.
See related posts:
Comments